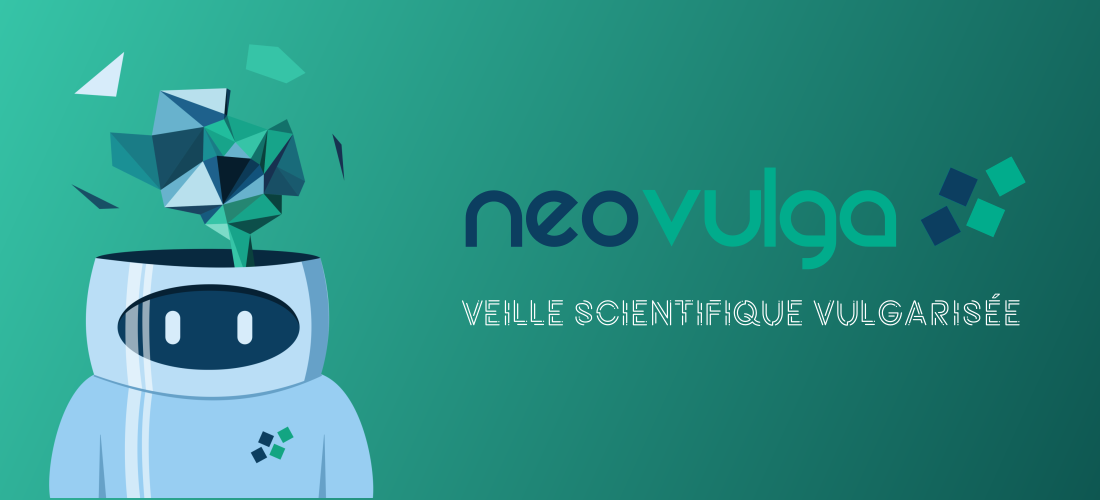
Neovulga – Vulgarized Knowledge – Detection of psychological diseases
Neovulga – Vulgarized Knowledge – Detection of psychological diseases
At Neovision, scientific monitoring is key to stay state of the art. Every month, the latest advances are presented to the entire team, whether it is new data sets, a new scientific paper… We screen almost all the news. In our ambition to make AI accessible to everyone, we offer you, every month, a simplified analysis of a technical topic presented by our R&D team.
Today, we will take a look at a scientific paper untitled Multimodal Deep Learning Framework for Mental Disorder Recognition, by Ziheng Zhang, Weizhe Lin, Mingyu Liu and Marwa Mahmoud.
Background
In 2017, the WHO estimated that more than 300 million people suffered from mental disorders. Disorders that can affect the well-being of an individual and their ability to work. For some people, mental disorders are also associated with a significant risk of mortality.
Currently, the method of detection of mental disorders is very subjective since it is based on clinical interviews and self-reported scores that can cause reporting errors. These errors cause a discrepancy between the individual’s declaration and reality.
There is therefore a need to develop a technology capable of automatically detecting and recognizing these disorders through early detection of symptoms, which would be particularly useful for preventing potential relapses. It would also provide information on the biological markers of the different mental disorders, thus facilitating their diagnosis.
However, establishing a diagnosis based on automated recognition implies taking into account several modalities, such as facial expressions, gestures, acoustic characteristics or verbal content. Indeed, an isolated modality rarely provides complete information and each one has its added value.
Presented breakthrough
The paper highlights a solution based on Deep Learning allowing to process individual multimodal features (visual, textual, auditory) by correlating them.
For this purpose, an autoencoder (more precisely a Multimodal Deep Denoising Autoencoder (multiDDAE)) is used to obtain multimodal representations of audiovisual features.
On the textual data side, a Paragraph Vector (PV) is used to process the transcribed interview documents containing clues about the presence of mental disorders.
The fusion of the textual and audiovisual information is collected and then placed in a MultiTask Deep Neural Network (DDN), which can adapt to different tasks to classify the pathology.
Note that the experimental evaluation focused on the recognition of bipolar disorder and depression.
Why is it awesome ?
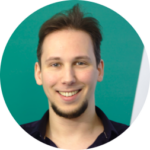
Arthur’s editorial
“Multimodal frameworks allow an AI method to address a problem using several different sources (audio, video, text…). In the case of diagnosing complex diseases such as mental illness, this approach is particularly well suited. Here, the authors have developed a multimodal network that can be easily adapted to the detection of different mental pathologies.”
This paper shows a great advance in the detection of mental disorders. Thanks to the experiments carried out on bipolarity and depression, the authors now know that their system is just as effective as the current state of the art on these two pathologies. This is very encouraging for the scientific community.
The major advantage of this solution is that it will allow a large number of specialists to detect symptoms earlier and to assist them in the diagnosis of mental illnesses. As the learning was done with a multimodal representation, the solution can be generalized to different types of mental disorders.
By expanding the datasets and continuing the training on other diseases, AI will be truly be a breakthrough for psychiatric diagnostic assistance.