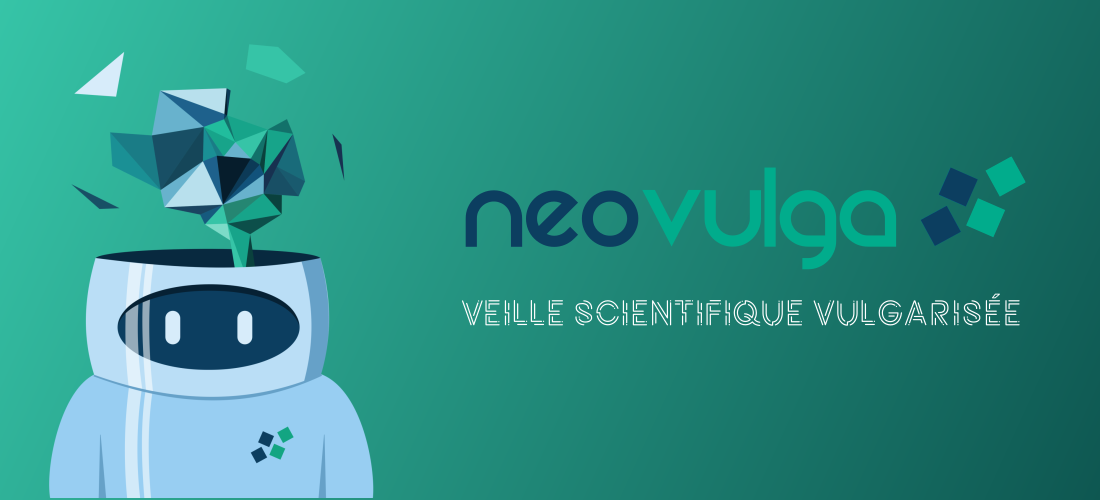
Neovulga – Vulgarized Knowledge – Holistic 3D Scene Understanding
Neovulga – Vulgarized Knowledge – Holistic 3D Scene Understanding
At Neovision, scientific monitoring is key to stay state of the art. Every month, the latest advances are presented to the entire team, whether it is new data sets, a new scientific paper… We screen almost all the news. In our ambition to make AI accessible to everyone, we offer you, every month, a simplified analysis of a technical topic presented by our R&D team.
Today we will take a look at the scientific paper Holistic 3D Scene Understanding from a Single Image with Implicit Representation, by Cheng Zhang, Zhaopeng Cui, Yinda Zhang, Bing Zeng, Marc Pollefeys, Shuaicheng Liu.
Background
The understanding of indoor 3D scenes is a complex problem. Indeed, the existing methods do not allow to elaborate precise estimates of the scene and the arrangements of the various objects. This is particularly due to strong occlusions between them.
Here, we propose a holistic method that combines different modules in order to perform accurate 3D reconstruction of indoor scenes from a single image.
Presented breakthrough
While classical methods require at least 2 images to estimate the depth and the layout of a room, here a single image is enough to generate a 3D reconstruction.
The architecture of the algorithm is made of different modules, each assigned to a specific task. One of them is in charge of estimating the plan of the room, i.e. the position of the different walls. Another one places bounding boxes around the objects. Finally, the last one makes a 3D segmentation of the objects and their shapes. It uses implicit functions for this.
These three modules make a first rough estimate of the different elements above. They create latent representations: they are not concrete poses, but they have a meaning for the network.
Then, a convolutional graph network refines the predictions. This type of network is very popular. It is based on a graph representation to make its calculations.
Graphs are representations with nodes connected to each other by links. Here, each object is a node and links connect the different objects. This makes it possible to say, for example, that the table is lies between the sofas, or that the frame is up on the wall above one of them.
Why is it awesome ?
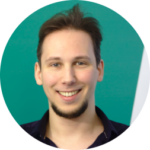
Arthur’s editorial
“Traditionally, 3D reconstruction involves using several images or various sensors in order to estimate the depth of what is perceived. In the same way for example that we use the parallax of our two eyes to perceive this information. But more and more, methods try to train networks to make this reconstruction from a single image.
This is the case here. But in addition to that, this article tries to reconstruct all the information of a scene at the same time: room plan, shape and orientation of the furniture and layout. This holistic method, by combining all these different state-of-the-art modules, achieves excellent performance.”
Here, the proposed method does not use any new technique. However, the combination between the different modules is done in a rather intelligent way and thus allows to go beyond the state of the art.
The input system requires only a single 2D image, and is therefore not dependent on a sensor or camera system. In addition, the holistic operation allows to use the different modules simultaneously. One can then do everything at once: identify the position of walls, position objects according to the right dimensions etc…
Small particularity, the network is composed of a cost function on the physical rules. This means that it will be penalized for any action performed that is physically impossible. For example, choosing to put the table and the sofa in the same place would be totally illogical.
There are several applications of 3D scene reconstruction. The most obvious one is augmented reality. One can imagine an interior designer creating rooms with different furniture layouts thanks to a complete analysis of the room.