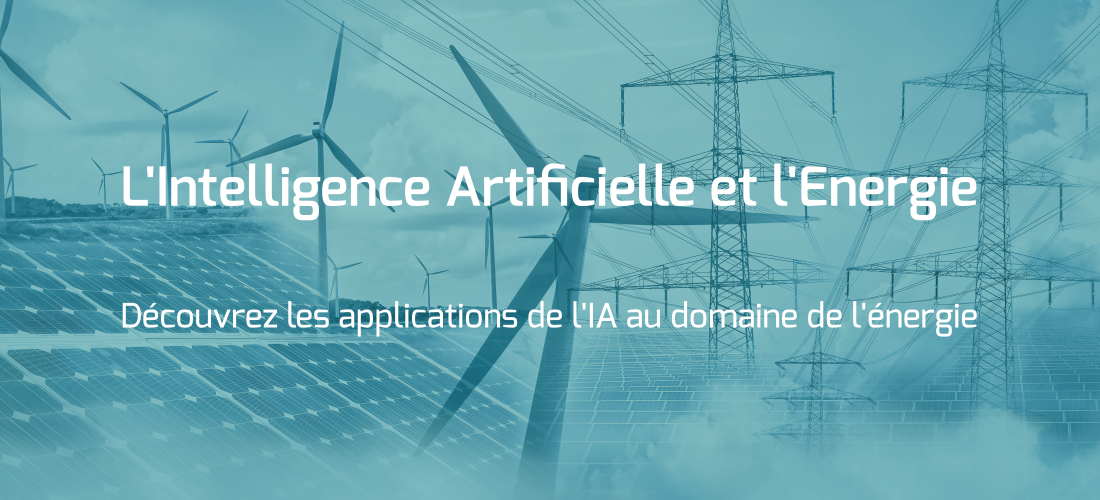
Artificial intelligence and energy
ARTIFICIAL INTELLIGENCE IN THE FIELD OF ENERGY
Artificial Intelligence has its share of enthusiasts and opponents. One of the favorite arguments of the latter is that AI, and more broadly digital technology, consumes a lot of energy and therefore has a huge environmental impact.
To deny the fact that machine learning is energy intensive would be lying straight to your face, and that doesn’t sound like Neovision.
However, while learning consumes energy, not all training is created equal. If GPT-3’s training is the carbon equivalent of a round-trip flight from New York to San Francisco, this is a pretty unique model and probably the heaviest in the world. It has ingested over 500 billion words, 150 times Wikipedia in all languages!
Moreover, if learning is energy-consuming, the situation is not the same for inference, that is to say when an AI is put into production and actually used.
To sum up, AI consumes a lot of energy for learning, but once it is in production, it consumes much less. Besides, learning is punctual, when inference is recurrent.
A bit like for humans, learning requires a lot of energy, but when we have a skill it is easy to use it regularly.
The following examples will show you that in the field of smart building or energy management, artificial intelligence has as many applications as benefits. Here’s a quick overview!
Smartbuilding takes care of your devices and your energy bill
What do you think is the most energy-intensive sector in France? We gave you a clue in the title. It is indeed the building industry! It alone represents 44% of the energy consumption in France. Without being really surprising, this observation invites us to question the importance of making our buildings less energy consuming.
If we can, of course, question certain habits and behaviors, artificial intelligence can also help us and accelerate the process.
By coupling the analysis of the energy consumption of certain equipment with the analysis of our habits and behaviors, an intelligent building is able to anticipate our needs, limit technological friction and smooth out our energy consumption.
For example, if a sensor indicates that there is no one left in a room on a Friday evening at 5pm and the history tells us that energy consumption decreases from 4:30pm, an artificial intelligence will be able to automate the BMS and thus turn off the lights and the air conditioning/heating that were left on. The result is savings on the bill and a reduced environmental impact! Jackpot!
Client case
Schneider and Neovision worked on this topic. The goal was to make an infrared sensor smart. By smart, we mean to be able to detect humans. So far, nothing revolutionary. But that was not all. In addition to detecting humans, the sensor also had to be able to recognize the postures of different people in the room: sitting, standing or lying down.
From this posture recognition, it was then necessary to deduce an activity. This was made possible thanks to machine learning.
The issues inherent to the sensor and its deployment were another challenge. To remain easy to deploy and install, the sensor had to be battery-powered. And the battery had to last several years. So the power and energy available was scarce.
In the end, the predictions produced by the Neovision AI were used to control the building’s BMS and thus optimize it: air conditioning, lighting, heating. The goal was to reduce and smooth out the building’s energy consumption!
Prediction and load shedding: goodbye to consumption peaks
Often presented as an ecological solution, electric cars also pose problems. The Chinese government can confirm that. Since 2017, the government has been encouraging its citizens to get electric cars and imposing impressive quotas on carmakers: to sell 12% electric vehicles by 2020.
But there is one issue. These large sales of electric cars are causing monumental spikes in consumption. Why? Because everyone is charging their cars at the same time. To meet this demand, coal-fired power plants are firing on all cylinders, causing CO2 emissions to skyrocket. The consequences are catastrophic with a large increase in air pollution.
Here again, AI has a role to play. The promotion of load shedding is an excellent solution to avoid consumption peaks and therefore pollution. To do this, it is necessary to be able to predict electricity consumption, in a fine and fast way, as close as possible to real time.
By predicting the real consumption a few hours in the future, it is possible to encourage the population or companies to reduce their electricity consumption and thus postpone certain non-urgent tasks by a few hours. By coupling this consumption prediction to a planning technology, industrial processes gain in flexibility, smooth out electricity consumption and allow society to save money by consuming when energy is cheaper.
When AI limits energy consumption… of AI!
As we know, artificial intelligence can consume a lot of energy – especially during the learning phase – this is a fact. Nevertheless, this is not inevitable thanks to AI. Artificial intelligence allows artificial intelligence to become more energy efficient (WTF?)
Today, data centers represent 1 to 3% of the world’s electricity consumption. This is both small and huge. A phenomenon that is expected to grow to 10% of global production by 2030! Other studies tend to show that the appetite of data centers is growing less rapidly than their capacities (+550% on the amount of computing between 2010 and 2018 while consumption has only increased by 6%).
Anyway, this is not nothing and it is easy to understand why energy efficiency is an issue that the GAFAs intend to tackle head on. To do so, they base their analyses on an index: the PUE (Power Usage Effectiveness). This index is the ratio between the total energy used by the data center and the energy actually consumed by the IT equipment. A PUE of 1 means that all the energy consumed is dedicated to the equipment: something impossible, but why? When IT equipment is running at full capacity, it produces heat and therefore needs cooling. And cooling machines requires energy. Most of the time, data centers rely on watercooling. To put it simply, pipes filled with fresh water flow through the servers to create a heat exchange.
Since 2014, Google has relied on AI to optimize its cooling system. In 2016, this artificial intelligence, developed by its subsidiary DeepMind, recommended an ideal operating scenario based on two predictions: temperatures and the workload demanded of servers.
But they went further. As early as 2018, we learned that now AI is driving the cooling of data centers in a completely autonomous way, without any human intervention. However, control mechanisms have been implemented so that humans can take over at any time!
But Google and other GAFAs are not the only ones working on this issue. Schneider Electric offers Cooling Optimize, an AI dedicated to driving air conditioning cabinets optimizing the airflow in a data center according to environmental conditions and changes in the infrastructure.